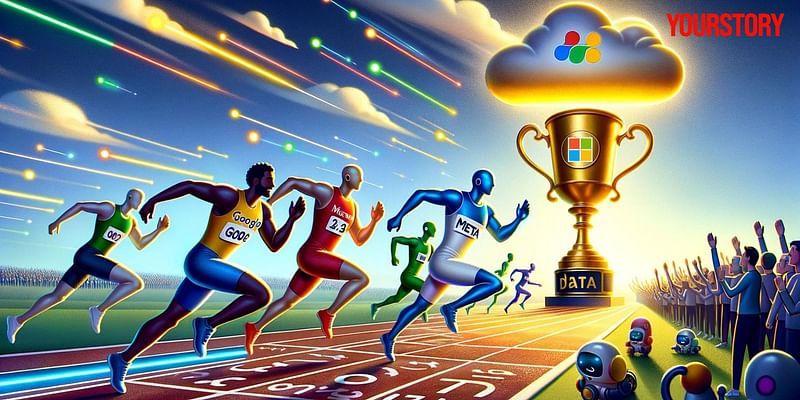
Artificial intelligence (AI) is no longer science fiction – it’s shaping our reality. From virtual assistants ordering groceries to self-driving cars navigating city streets, AI is rapidly transforming how we live and work. But just like a car needs fuel to run, AI needs data – vast amounts of it – to learn and perform its magic. This is where the plot thickens, and Big Tech companies are in a heated race to buy up all the AI training data they can find. Let’s decode this data dilemma and see why information is the new gold in the age of AI.
Why Data is the Secret Sauce for AI Success
Imagine teaching a child to recognise a dog. You wouldn’t show them just one picture, right? The more images they see, the better they’ll understand the concept. AI works similarly. It learns from massive datasets of text, images, videos, and other information. The more data it has to crunch, the better it performs. This is where Big Tech enters the scene. Companies like Google, Microsoft, and Facebook generate a mountain of data from user interactions, searches, and online activity. This data is a goldmine for training AI models that can:
- Craft hyper-personalised experiences: Imagine a shopping website that anticipates your needs before you even type them in. That’s the power of AI trained on vast amounts of user data.
- Revolutionise industries: From healthcare diagnostics to financial forecasting, AI trained on relevant data sets can unlock groundbreaking advancements across various sectors.
- Push the boundaries of language processing: Machine translation that feels natural, chatbots that hold engaging conversations – these are all within reach with the right AI training data.
The Data Dilemma: Not All Data is Created Equal
While Big Tech has a data advantage, quality matters more than quantity. Here’s where the challenge lies:
- Bias Blues: Data reflecting real-world biases can lead to AI models perpetuating those biases. For example, an AI trained on biased news articles might struggle to deliver objective results.
- Data Scarcity: Specific types of data, like medical records or financial transactions, might be scarce due to privacy concerns. This creates a bottleneck for developing AI solutions in certain fields.
- The Ethical Maze: The question of data ownership and privacy is paramount. Who owns the data we generate online, and how can it be used ethically for AI development?
Beyond the Bidding Wars: Alternative Data Solutions
The Big Tech data buying frenzy isn’t the only solution. Here are some emerging trends:
- Synthetic Data Generation: Creating realistic simulated data can address scarcity issues and mitigate bias concerns.
- Data Crowdsourcing: Incentivising individuals to contribute specific types of data can help fill knowledge gaps.
- Focus on Data Anonymisation: Techniques that anonymise data while preserving its utility can be a win-win for both AI development and user privacy.
The Future of AI: A Collaborative Dance with Data
The race for AI training data isn’t just about who has the most. It’s about who can leverage data responsibly, ethically, and creatively. Collaboration between Big Tech, research institutions, and data privacy advocates is crucial.
By fostering a responsible approach to data collection and utilisation, we can ensure that AI continues to evolve for the benefit of everyone, not just the highest bidder. The future of AI is bright, but it depends on a healthy relationship with the data that fuels it. Let’s make sure this dance with data leads to a future filled with innovation, not exploitation.
Edited by Rahul Bansal