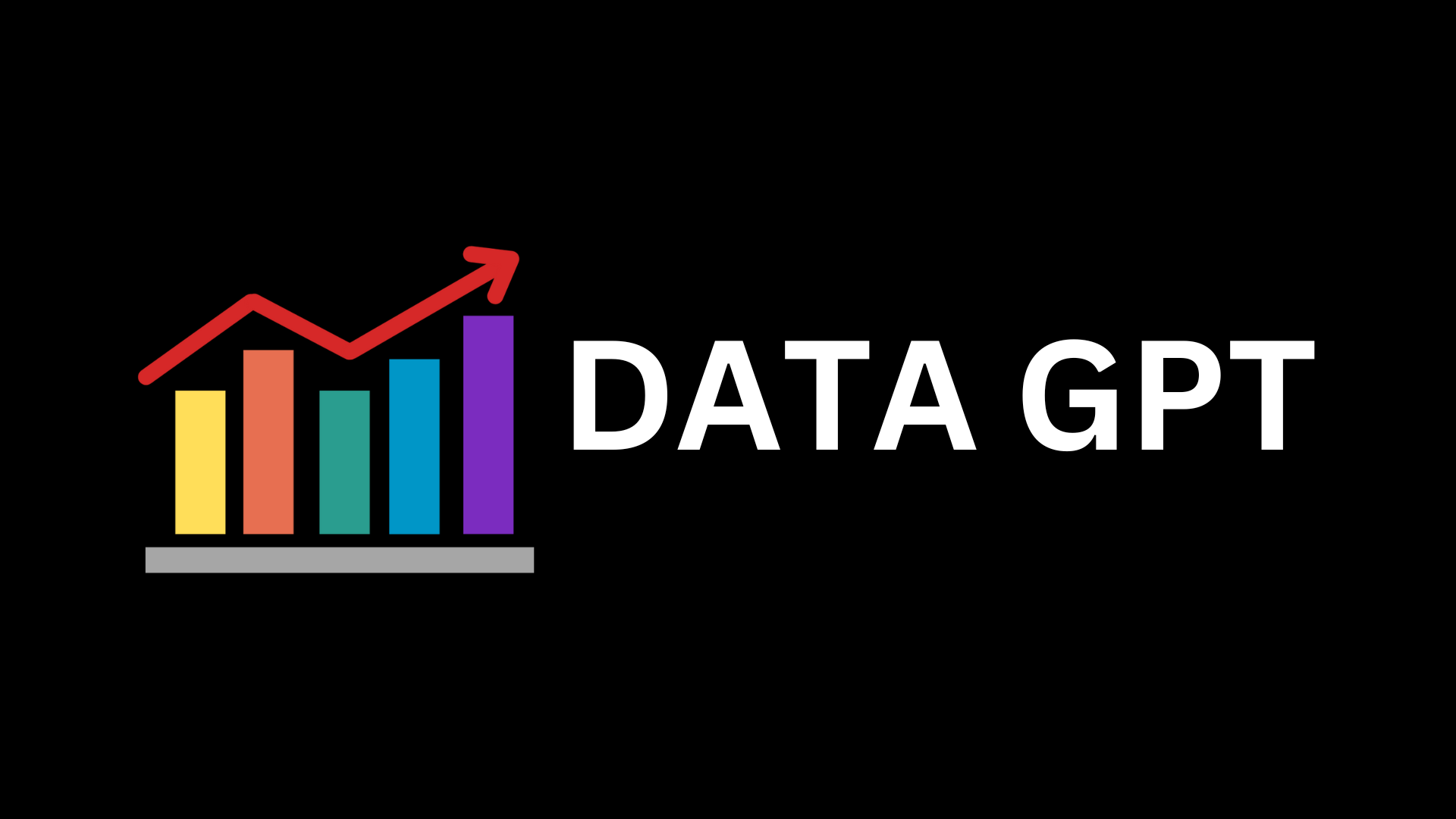
California-based startup DataGPT has emerged from stealth mode, unveiling its new AI Analyst, which leverages conversational AI to help enterprises extract insights from their data.
The AI Analyst integrates language understanding capabilities with a proprietary analytics engine, enabling users to communicate with their data in natural language.
This article explores how DataGPT’s AI Analyst works and the benefits it offers to enterprises.
How does DataGPT’s AI analyst work?
DataGPT’s AI Analyst simplifies the process of obtaining insights from data by offering a chatbot-style conversational experience. It comprises two key elements:
AI analyst
This is the chatbot component that allows users to input questions in natural language. It processes queries and provides immediate access to relevant insights.
Data navigator
This component offers a more traditional approach, displaying visualisations of key metrics‘ performance and allowing users to manually explore data through various factors.
Key differentiation
DataGPT claims to differentiate itself with the analytical prowess of its engine. It argues that other solutions either focus on language models with limited data volumes or integrate generative AI into traditional BI workflows, resulting in narrow reports and dashboards.
DataGPT’s approach combines contextual comprehension from language models with deep data analysis and interpretation, offering a more comprehensive and user-friendly data experience.
How does DataGPT benefit enterprises?
Enterprises that have adopted DataGPT’s AI Analyst have reported several benefits:
Accelerated insights
The chatbot-style interface speeds up the process of obtaining insights, helping businesses make critical decisions more quickly.
Time savings
DataGPT frees up analysts’ time by automating data queries and analysis, allowing them to focus on more strategic tasks.
Performance
DataGPT’s lightning cache database is significantly faster than traditional databases, running queries up to 600 times faster than standard business intelligence tools.
Cost efficiency
It reduces the cost of data analysis by up to 15 times, providing cost-effective insights.
Revenue growth
The insights made possible by DataGPT can unlock up to 15% revenue growth for businesses.
The 3 layers of the AI Analyst
Data store
It stores the data schema, enabling the system to understand the data structure and relationships.
Core analytics engine
This component executes analysis tasks, including computing impacts, employing statistical tests, and calculating confidence intervals. It runs thousands of queries in a lightning-fast cache of the data store.
Analyst agent
This part is powered by a self-hosted large language model (LLM). When users ask questions, this LLM creates a task plan based on the query and then sends each task to the Core Analytics Engine. The results are delivered in a conversational format to the user.
The combination of these elements allows users to interact with their data in a user-friendly and conversational manner, bridging the gap between traditional reports and informed decision-making
Future plans for DataGPT
DataGPT, which has raised $10 million in pre-seed and seed rounds, aims to expand its analytical capabilities. Future enhancements include cohort analysis, forecasting, and predictive analysis.
While the exact timeline for these capabilities is not disclosed, DataGPT plans to remain competitive in a market where many data ecosystem vendors are integrating generative AI into their offerings.
Embrace the future of data analysis with DataGPT’s AI Analyst. It’s not just about understanding data; it’s about having a conversation with it.