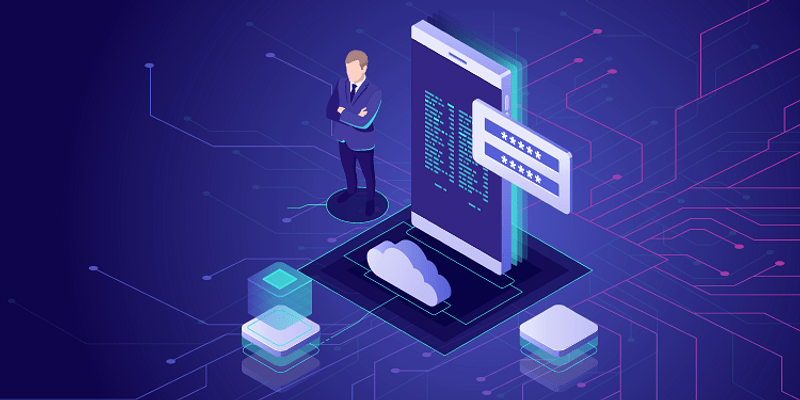
The synergy of AI and cloud computing produces unmatched potential for companies leaning towards agile solutions. According to a recent report, the global cloud AI market is forecasted to grow to $215.39 billion by 2027. This dynamic fusion is launching revolutionary AI cloud solutions, making a dent in the digital landscape.
As advanced large language model (LLM) tools like Google Bard (now Gemini) and ChatGPT gain popularity, AI cloud computing is essential in shaping data collection and management methods, enabling strategic decision-making in cloud environments.
Indeed, with their unique capabilities, AI cloud solutions are the talk of the town, but they are not without challenges. Here are some of the challenges of integrating AI in cloud computing:
Data privacy, security and compliance challenges
One of the primary challenges of AI and cloud computing integration is the security of the sheer data volume. While AI chatbots like ChatGPT and Bing have become the one-stop solutions for individuals and organisations, concerns regarding data security are turning heads. Such tools require and use extensive datasets to train and upgrade continuously, which raises serious issues related to privacy and information security.
Moreover, regulatory challenges come hand-tied with data handling. The problem is far more intense for multinational companies dealing with restrictions on international data transfer. Complying with digital regulations across verticals while ensuring seamless cloud operations is often easier said than done.
Solution: To fuel this growing synergy of AI and cloud computing and continue reaping the benefits of technological advancements, it’s essential to be mindful of the security policies of the various LLMs. Besides, strong cybersecurity measures must also be implemented to prevent security breaches. To address the regulatory challenges, companies must implement data validation, consistency, accuracy, and quality checks at each level of the integration process. An internal data governance setup that includes data lineage, pipelines, audit trails, and metadata management is required.
As more AI cloud computing solutions are implemented, adopting an AI risk management framework is essential to protect individuals, organisations, and society. Considering this objective, the EU regulated the first comprehensive AI law as part of its digital strategy. CapAI, an ethics-based auditing method, provides another precaution against possible AI security threats. This procedure conducts conformity tests of AI technologies in line with unethical anomalies in AI infrastructures.
Data management
Siloed data within different departments or cloud platforms hinders seamless integration. However, connecting these diverse data is challenging since AI models and cloud services may not seamlessly communicate, resulting in interoperability challenges. Furthermore, standardised formats and protocols for data exchange are often absent, which makes managing these data and using the same to train AI models complex.
Solution: The solution lies in using integration platforms that act like super connectors, linking various cloud services and AI tools seamlessly. Besides using standardised data formats and protocols, companies must keep their APIs well-documented and abide by the industry-prescribed best practices to enhance interoperability.
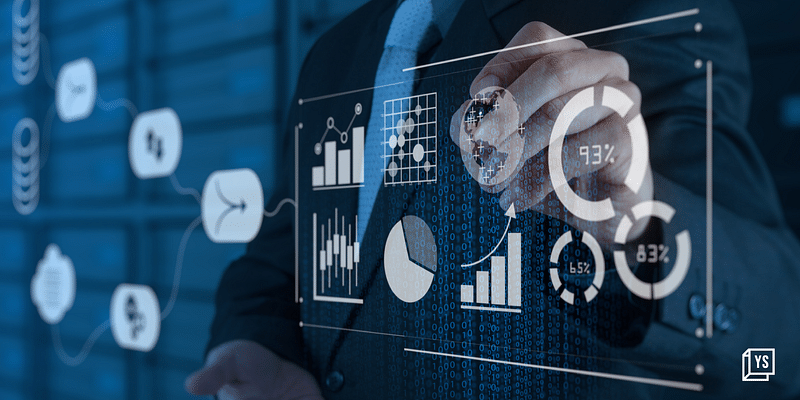
.thumbnailWrapper
width:6.62rem !important;
.alsoReadTitleImage
min-width: 81px !important;
min-height: 81px !important;
.alsoReadMainTitleText
font-size: 14px !important;
line-height: 20px !important;
.alsoReadHeadText
font-size: 24px !important;
line-height: 20px !important;
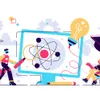
Model training and deployment
Efficient model training and deployment in a cloud-native environment can be complex. Model training is a resource-intensive process requiring meticulous planning and implementation. Additionally, managing the various distributed systems to maintain consistency makes the operation even more intricate. Though essential, keeping track of the different models and code versions is difficult due to frequent updates and changes. Furthermore, while orchestration tools are used for managing cloud-native applications, integrating machine learning models into containerised environments adds an extra layer of complexity.
Solution: Tackling issues in model training becomes easy using versioning techniques. These methods, like A/B testing, allow brands to test various deployed models with easy rollback options if needed. Traffic management tools are also helpful.
While dealing with diverse distributed systems, data-parallel training is a sought-after solution. Training techniques like TensorFlow’s Distributed Training or PyTorch’s Distributed Data Parallelism manage various distributed systems consistently.
Regarding orchestration, it is advisable to use microservices clubbed with automated CI/CD pipelines to ensure seamless container orchestration.
Finally, brands can automate the model selection and tuning process using AutoML tools/services like Google AutoML and Azure AutoML to streamline workflow.
Cost management challenge
AI cloud computing solutions, especially ones involving deep learning, are resource-intensive. These models consume thousands of Graphics Processing Units (GPUs) to handle the vast database they learn from. Additionally, these models require multiple training iterations for development and fine-tuning, which adds to the final costs. According to analysts, the training costs for large language models like ChatGPT can cost $4 million or more!
Also, network latency affects the costly training process, resulting in slow integration.
Solution: Thinking about costs is important as AI transforms industries. When deciding whether to create or improve an AI LLM, companies should think about things like how much time and money they have, the quality and amount of data they have, how skilled their team is with technology, and whether the AI strategy fits with their overall business plan. These factors help make smart decisions about whether to build a new model or improve an existing one.
.thumbnailWrapper
width:6.62rem !important;
.alsoReadTitleImage
min-width: 81px !important;
min-height: 81px !important;
.alsoReadMainTitleText
font-size: 14px !important;
line-height: 20px !important;
.alsoReadHeadText
font-size: 24px !important;
line-height: 20px !important;
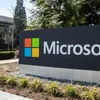
Observability in AI
While integrating AI in cloud environments powers advantages like scalability and efficient resource use, monitoring and observability are more challenging, resulting in manageability issues. The challenge resonates with the tendency of LLMs like ChatGPT to “hallucinate” or, in other words, make things up. Since AI apps use machine learning to process data instead of using computer codes, the ability to interpret an application’s outcome becomes unpredictable. From the observability perspective, traditional debugging and troubleshooting often don’t function for such apps, making it difficult to determine the number of calls, request size, and more.
Solutions: A logging and monitoring system will provide a comprehensive view of the inputs and outputs and assess the overall health of the AI model. Additionally, a distributed tracking facility can make a route map of the request flows within the AI cloud environment. These solutions boost relevant data collection, determine system functions, monitor resource utilisation, and predict AI behaviour patterns.
Lack of tech skill
The skill gap has reached a concerning level. EY’s ‘Navigating the future of work in 2025 and beyond’ report notes that 81% of organisations are experiencing a tech talent shortage. This problem has profound implications for AI cloud computing integration. Combining and deploying AI cloud services demands advanced skills that are scarce in the current workforce. Though companies opt for AI cloud computing solutions, most need more in-house talent to execute AI initiatives.
Solution: Upskilling and using new-age tech to solve real-life business challenges is the best way to bridge the skill gap. Companies must invest in staff training through online resources or collaborate with cloud vendors and external consultants to overcome the talent shortage challenge.
(Mayank Mishra is the VP of Engineering at Contentstack, a composable digital experience platform.)
Edited by Kanishk Singh
(Disclaimer: The views and opinions expressed in this article are those of the author and do not necessarily reflect the views of YourStory.)